Program verification is making considerable progress but is hampered by a lack of abstraction in specifications. A crucial step is, almost always, absent from the process; this omission is the principal obstacle to making verification a standard component of everyday software development.
1. Steps in software verification
In the first few minutes of any introduction to program verification, you will be told that the task requires two artifacts: a program, and a specification. The program describes what executions will do; the specification, what they are supposed to do. To verify software is to ascertain that the program matches the specification: that it does is what it should.
The consequence usually drawn is that verification consists of three steps: write a specification, write a program, prove that the program satisfies the specification. The practical process is of course messier, if only because the first two steps may occur in the reverse order and, more generally, all three steps are often intertwined: the specification and the program influence each other, in particular through the introduction of “verification conditions” into the program; and initial proof attempts will often lead to changes in both the specification and the program. But by and large these are the three accepted steps.
Such a description misses a fourth step, a prerequisite to specification that is essential to a scalable verification process: Domain Theory. Any program addresses a specific domain of discourse, be it the domain of network access and communication for a mobile phone system, the domain of air travel for a flight control system, of companies and shares for a stock exchange system and so on. Even simple programs with a limited scope, such as the computation of the maximum of an array, use a specific domain beyond elementary mathematics. In this example, it is the domain of arrays, with their specific properties: an array has a range, a minimum and maximum indexes in that range, an associated sequence of values; we may define a slice a [i..j], ask for the value associated with a given index, replace an element at a given index and so on. The Domain Theory provides a formal model for any such domain, with the appropriate mathematical operations and their properties. In the example the operations are the ones just mentioned, and the properties will include the axiom that if we replace an element at a certain index i with a value v then access the element at an index j, the value we get is v if i = j, and otherwise the earlier value at j.
2. The role of a Domain Theory
The task of devising a Domain Theory is to describe such a domain of reference, in the spirit of abstract data types: by listing the applicable operations and their properties. If we do not treat this task as a separate step, we end up with the kind of specification that works for toy examples but quickly becomes unmanageable for real-life applications. Most of the verification literature, unfortunately, relies on such specifications. They lack abstraction since they keep using the lowest-level mathematical objects and constructs, such as numbers and quantified expressions. They are to specification what assembly language is to modern programming.
Dines Bjørner has for a long time advocated a closely related idea, domain engineering; see for example his book in progress [1]. Unfortunately, he does not take advantage of modularization through abstract data types; the book is an example of always-back-to-the-basics specification, resorting time and again to fully explicit specifications based on a small number of mathematical primitives, and as a consequence making formal specification look difficult.
3. Maximum computed from both ends
As a simple example of modeling through an abstract theory consider an algorithm for computing the maximum of an array. We could use the standard technique that goes through the array one-way, but for variety let us take the algorithm that works from both ends, moving two integer cursors towards each other until they meet. (This example was used in a verification competition at a recent conference, I forgot which one.) The code looks like this:
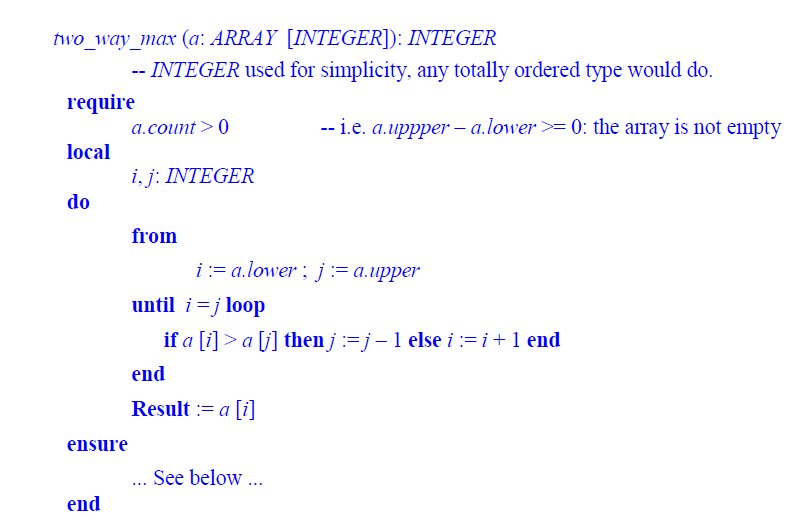
The specification, expressed by the postcondition (ensure) should state that Result is the maximum of the array; the loop invariant will be closely related to it. How do we express these properties? The obvious way is not the right way. It states the postcondition as something like
∀ k: Z | (k ≥ a.lower ∧ k ≤ a.upper) ⇒ a [k] ≤ Result
∃ k: Z | k ≥ a.lower ∧ k ≤ a.upper ∧ a [k] = Result
In words, Result is at least as large as every element of the array, and is equal to at least one of the elements of the array. The invariant can also be expressed in this style (try it).
The preceding specification expresses the desired property, but it is of an outrageously lower level than called for. The notion of maximum is a general one for arrays over an ordered type. It can be computed through many different algorithms in addition to the one shown above, and exists independently of these algorithms. The detailed, assembly-language-like definition of its properties should not have to be repeated in every case. It should be part of the Domain Theory for the underlying notion, arrays.
4. A specification at the right level of abstraction
In a Domain Theory for arrays of elements from an ordered set, one of the principal operations is maximum, satisfying the above properties. The definition of maximum through these properties belongs at the Domain Theory level. The Domain Theory should include that definition, independent of any particular computational technique such as two_way_max. Then the routine’s postcondition, relying on this notion from the Domain Theory, becomes simply
Result = a.maximum
The application of this approach to the loop invariant is particularly interesting. If you tried to write it at the lowest level, as suggested above, you should have produced something like this:
a.lower ≤ i ≤ j ≤ a.upper
∃ k: Z | k ≥ i ∧ k ≤ j ∧ (∀ l: Z | l ≥ a.lower ∧ l ≤ a.upper ⇒ a [l] ≤ a [k])
The first clause is appropriate but the rest is horrible! With its nested quantified expressions it gives an impression of great complexity for a property that is in fact straightforward, simple enough in fact to be explained to a 10-year-old: the maximum of the entire array can be found between indexes i and j. In other words, it is also the maximum of the array slice going from i to j. The Domain Theory will define the notion of slice and enable us to express the invariant as just
a.lower ≤ i ≤ j ≤ a.upper — This bounding clause remains
a.maximum = (a [i..j ]).maximum
(where we will write the slice a [i..j ] as a.slice (i, j ) if we do not have mechanisms for defining special syntax). To verify the routine becomes trivial: on loop exit the invariant still holds and i = j, so the maximum of the entire array is given by the maximum of the single-element slice a [i..i ], which is the value of its single element a [i ]. This last property — the maximum of a single-element array is its single value — is independent of the verification of any particular program and should be proved as a little theorem of the Domain Theory for arrays.
The comparison between the two versions is striking: without Domain Theory, we are back to the most tedious mathematical manipulations again and again; simple, clear properties look complicated and obscure. This just for a small example on basic data structures; now think what it will be for a complex application domain. Without a first step of formal modeling to develop a Domain Theory, no realistic specification and verification process is realistic.
Although the idea is illustrated here through examples of individual routines, the construction of a Domain Theory should usually occur, in an object-oriented development process, at the level of a class: the embodiment of an abstract data type, which is at the appropriate level of granularity. The theory applies to objects of a given type, and hence will be used for the verification of all operations of that type. This observation justifies the effort of devising a Domain Theory, since it will benefit a whole set of software elements.
5. Components of a Domain Theory
The Domain Theory should include the three ingredients illustrated in the example:
- Operations, modeled as mathematical functions (no side effects of course, we are in the world of specification).
- Axioms characterizing the defining properties of these operations.
- Theorems, characterizing other important properties.
This approach is of course nothing else than abstract data types (the same thing, although few people realize it, as object-oriented analysis). Even though ADTs are a widely popularized notion, supported for example by tools such as CafeOBJ [2] and Maude [3], it is generally not taken to its full conclusions; in particular there is too often a tendency to define every new ADT from scratch, rather than building up libraries of reusable high-level mathematical components in the O-O spirit of reuse.
6. Results, not just definitions
In devising a Domain Theory with the three kinds of ingredient listed above, we should not forget the last one, the theorems! The most depressing characteristic of much of the work on formal specification is that it is long on definitions and short on results, while good mathematics is supposed to be the reverse. I think people who have seriously looked at formal methods and do not adopt them are turned off not so much by the need to use mathematics but by the impression they get little value for it.
That is why Eiffel contracts do get adopted: even if it’s just for testing and debugging, people see immediate returns. It suffices for a programmer to have caught one bug as the violation of a simple postcondition to be convinced for life and lose any initial math-phobia.
7. Quantifiers are evil
As we go beyond simple contract properties — this argument must be positive, this reference will not be void — the math needs to be at the same level of abstraction to which, as modern programmers, we are accustomed. For example, one should always be wary of program specifications relying directly on quantified expressions, as in the low-level variants of the postcondition and loop invariant of the two_way_max routine.
This is not just a matter of taste, as in the choice in logic [4] between lambda expressions (more low-level but also more immediately understandable) and combinators (more abstract but, for many, more abstruse). We are talking here about the fundamental software engineering problem of scalability; more generally, of the understandability, extendibility and reusability of programs, and the same criteria for their specification and verification. Quantifiers are of course needed to express fundamental properties of a structure but in general should not directly appear in program assertions: as the example illustrated, their level of abstraction is lower than the level of discourse of a modern object-oriented program. If the rule — Quantifiers Considered Harmful — is not absolute, it must be pretty close.
Quantified expressions, “All elements of this structure possess this property” and “Some element of this structure possesses this property” — belong in the description of the structure and not in the program. They should appear in the Domain Theory, not in the verification. If you want to express that a hash table search found an element of key K, you should not write
(Result = Void ∧ (∀ i: Z | i ≥ a.lower ∧ i ≤ a.upper ⇒ a.item (i).key ≠ K))
∨
(Result ≠ Void ∧ (∀ i: Z | i ≥ a.lower ∧ i ≤ a.upper ∧ a.item (i).key = K ∧ Result = a.item (i))
but
Result /= Void ⇔ (Result ∈ a.elements_of_key (K))
The quantified expressions will appear in the Domain Theory for the corresponding structure, in the definition of such domain properties as elements_of_key. Then the program’s specification — the contracts to be verified — can rely on concepts that make sense to the programmer; the verification will take advantage of theorems that have been proved independently since they belong to the Domain Theory and do not depend on individual programs.
8. Even the simplest examples…
Practical software verification requires Domain Theory even in the simplest cases, including those often used as purely academic examples. Perhaps the most common (and convenient) way to explain the notion of loop invariant is Euclid’s algorithm to compute the greatest common divisor (gcd) of two numbers (with a structure remarkably similar to that of two_way_max):
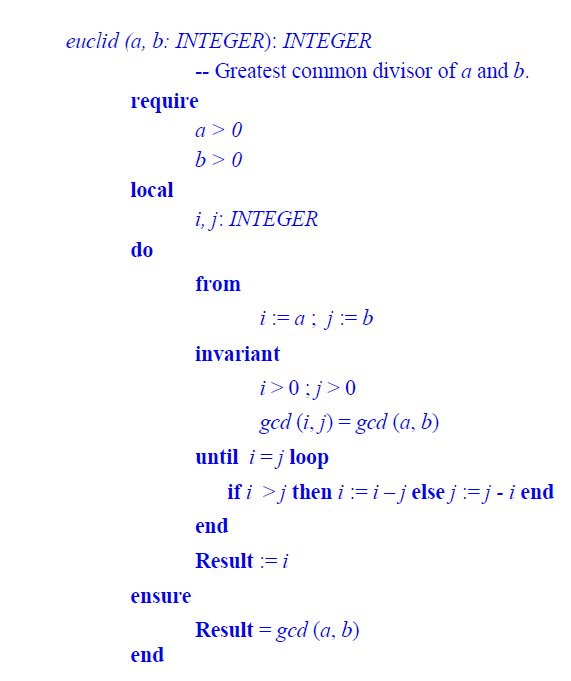
I have expressed the postcondition using a concept from an assumed Domain Theory for the underlying problem: gcd, the mathematical function that yields the greatest common divisor of two integers. Many specifications I have seen go back to the basics, with something like this (using \\ for integer remainder):
a \\ Result = 0 ∧ b \\ Result = 0 ∧ ∀ i: N | (a \\ i = 0) ∧ (b \\ i = 0) ⇒ i ≤ Result
This is indeed the definition of what it means for Result to be the gcd of a and b (it divides a, it divides b, and is greater than any other integer that also has these two properties). But it makes no sense to include such a detailed mathematical property in the specification of a program element. It belongs in the domain theory, where it will serve as the definition of a function gcd, which we can then use directly in the specification of the program.
Note how the invariant makes the necessity of the Domain Theory approach even more clear: try to express it in the basic mathematical form, not using the function gcd, It can be done, but the result is typical of the high complexity to usefulness ratio of traditional formal specifications mentioned above. Instead, the invariant that I have included in the program text above says exactly what there is to say, clearly and concisely: at each iteration, the gcd of our two temporary values, i and j, is the result that we are seeking, the gcd of the original values a and b. On exit from the loop, when i and j are equal, their common value is that result.
It is also thanks to the Domain Theory modeling that the verification of the program — consisting of proving that the stated property is indeed invariant — will be so simple: as part of the theory, we should have the two little theorems
i > j > 0 ⇒ gcd (i, j) = gcd (i –j, j)
gcd (i, i) = i
which immediately show the implementation to be correct.
Inside of any big, fat, messy, quantifier-ridden specification there is a simple, elegant and clear Domain-Theory-based specification desperately trying to get out. Find it and use it.
9. From Domain Theory to domain library
One of the reasons most people working on program verification have not used the division into levels of discourse described here, with a clear role for developing a Domain Theory, is that they lack the appropriate notational support. Mathematical notation is of course available, but we are talking about programs a general verification framework cannot resort to a new special notation for every new application domain.
This is one of the places where Eiffel provides a consistent solution, through its seamless approach to integrating programs and specifications in a single notation. Thanks to mechanisms such as deferred classes (classes that describe concepts through detailed specifications without committing to an implementation), Eiffel is as much for specification as for design and implementation; a Domain Theory can be expressed though a set of deferred Eiffel classes, which we may call a domain library. The classes in a domain library should not just be deferred, meaning devoid of implementation; they should in addition describe stateless operations only — queries, not commands — since they are modeling purely mathematical concepts.
An earlier article in this blog [5] outlined the context of our verification work: the EVE project (Eiffel Verification Environment), a practical approach to integrating software verification in the day-to-day practice of modern software development, with the slogan ““Verification As a Matter Of Course”. In this project we have applied the idea of Domain Theory by building a domain library covering fundamental concepts of set theory, including functions and relations. This is the Mathematical Model Library (MML) [6, 7], which we use to verify the new data structure library EiffelBase 2 using specifications at the appropriate level of abstraction.
MML is in fact useful for the specification of a wide variety of programs, since almost every application area can benefit from the general concepts of set, subset, relation and such. But to cover a specific application domain, say flight traffic control, MML will generally not suffice; you will need to devise a Domain Theory that mathematically models the target domain, and may express it in the form of a domain library written in the same general spirit as MML: all deferred, stateless, focused on high-level abstractions.
It is one of the attractions of Eiffel that you can express such a theory and library in the same notation as the programs that will use it — more precisely in a subset of that notation, since the specification classes do not need the imperative constructs of the language such as instructions and attributes. Then both the development process and the verification use a seamlessly integrated set of notations and techniques, and all use the same tools from a modern IDE, in our case EiffelStudio, for browsing, editing, working with graphical repreentation, metrics etc.
10. DSL libraries for specifications
A mechanism to express Domain Theories is to a general specification mechanism essentially like a Domain Specific Language (DSL) is to a general programming language: a specialization for a particular domain. Domain libraries make the approach practical by:
- Embedding the specification language in the programming language.
- Fundamentally relying on reuse, in the best spirit of object technology.
This approach is in line with the one I presented for handling DSLs in an earlier article of this blog [8] (thanks, by the way, for the many comments received, some of them posted here and some on Facebook and LinkedIn where the post triggered long discussions). It is usually a bad idea to invent a new language for a new application domain. A better solution is to rely on libraries, by taking advantage of the power of object-oriented mechanisms to model (in domain libraries) and implement (for DSLs) the defining features of such a domain, and to make the result widely reusable. The resulting libraries are purely descriptive in the case of a domain library expressing a Domain Theory, and directly usable by programs in the case of a library embodying a DSL, but the goal is the same.
11. A sound and necessary engineering practice
Many ideas superficially look similar to Domain Theory: domain engineering as mentioned above, “domain analysis” as widely discussed in the requirements literature, model-driven development, abstract data type specification… They all start from some of the same observations, but Domain Theory as described in this article is something different: a systematic approach to modeling an arbitrary application domain mathematically, which:
- Describes the concepts through applicable operations, axioms and (most importantly) theorems.
- Expresses these elements in an applicative (side-effect free, i.e. equivalent to pure mathematics) subset of the programming language, for direct embedding in program specifications.
- Relies on the class mechanism to structure the results.
- Collects the specifications into specification libraries and promotes the reuse of specifications in the same way we promote software reuse.
- Uses the combination of these techniques to ensure that program specifications are at a high level of abstraction, compatible with the programmers’ view of their software.
- Promotes a clear and effective verification process.
The core idea is in line with standard engineering practices in disciplines other than software: to build a bridge, a car or a chip you need first to develop a sound model of the future system and its environment, using any useful models developed previously rather than always going back to elementary textbook mathematics.
It seems in fact easier to justify doing Domain Analysis than to justify not doing it. The power of expression and abstraction of our programs has grown by leaps and bounds; it’s time for our specifications to catch up.
References
[1] Dines Bjørner: From Domains to Requirements —The Triptych Approach to Software Engineering, “to be submitted to Springer”, available here.
[2] Kokichi Futatsugi and others: CafeObj page, here.
[3] José Meseguer and others: Maude publication page, here.
[4] J. Roger Hindley, J. P. Seldin: Introduction to Combinators and l-calculus, Cambridge University Press, 1986.
[5] Verification As a Matter Of Course, earlier article on this blog (March 2010), available here.
[6] Bernd Schoeller, Tobias Widmer and Bertrand Meyer. Making specifications complete through models, in Architecting Systems with Trustworthy Components, eds. Ralf Reussner, Judith Stafford and Clemens Szyperski, Lecture Notes in Computer Science, Springer-Verlag, 2006, pages 48-70, available here.
[7] Nadia Polikarpova, Carlo A. Furia and Bertrand Meyer: Specifying Reusable Components, in VSTTE’10: Verified Software: Theories, Tools and Experiments, Edinburgh, August 2010, Lecture Notes in Computer Science, Springer-Verlag, available here.
[8] Never Design a Language, earlier article on this blog (January 2012), available here.
VN:F [1.9.10_1130]
Rating: 9.3/10 (12 votes cast)
VN:F [1.9.10_1130]
Rating: +7 (from 7 votes)